Author: Andrew Morgan, CFA
As data sets grow in critical mass can they be used to foretell the future? Andrew Morgan, CFA, investigates
We’ve all had that fantasy: venturing back in time to change your past decisions based on what you know today. Perhaps it’s a choice of lottery numbers, buying bitcoin before the frenzy, or even a particular stock that was unknowingly poised to skyrocket. But sadly, as the late Stephen Hawking had most likely proven, time travel seems to be out of our grasp for now.
Along the same vein, predicting the future would be another convenient talent for the asset management employee (provided you’re the only one who has it), but sadly also out of the realms of possibility.
However, many of us in the investment industry (and indeed across any industry) spend a lot of time trying to do exactly this. Whether you favour fundamental, technical, qualitative or quantitative methods, most managers and analysts are trying to find those magical correlations and causations to make a case for an investment decision, based on some future outcome one is trying to anticipate.
Of course this is notoriously difficult – if there is an obvious indicator then everyone will already know it and it will be priced in. It may be very subtle, and then the question is of timing and whether the indicator was indeed at all correlated, or perhaps merely down to luck (although no one would admit that).
Then there is the issue of those less scrupulous managers who just have a gut feeling, and analysts are controversially tasked to back this opinion up with data, going against any scientific and perhaps ethical principle. Or is something so at the mercy of the whims of mostly irrational participants, just impossible to predict. Whatever your view, the industry is built on information, and making use of that information to better understand the market.
Is alternative data the answer?
This is where the idea of alternative data is positioned to fundamentally change the investment process and profession. While not necessarily a new idea in some of the more scientifically based hedge funds, the landscape is in dramatic flux and ever expanding, opening up new datasets to explore with evolving methods using exponentially increasing computing capabilities.
What is alternative data? As the term suggests, this is data not yet commonly used in the investment decision. While many are well versed in traditional pricing models making use of metrics we learn in basic financial analysis, this alternative data makes use of much broader, unstructured and more loosely related non-financial factors, for example:
- Satellite images of car parks, construction sites and shipping routes to ascertain changes in economic activity
- Foot traffic outside retail shops, as a proxy for understanding sales growth
- Social media feeds, indicating sentiment of particular brands or more generally of the economy
- Search activity and trends, be it direct searches for companies, products or services indicating future sales, or even more subtle e.g. searches of health symptoms indicating an outbreak and therefore an upward trend in a certain drug company’s specialist products
For the more traditional investor, it may seem a bit farfetched that useful and useable investment signals could be discovered in such diverse data. Although, many quantitative and hedge funds have already been early adopters of these methods (see figure 1), we are still in the early stages of adoption. By some accounts we are in the ‘Peak of inflated Expectations’ (figure 2) due to the general hype around artificial intelligence and some datasets have found to have little or no signal. However, this is likely to change as data sets grow and gain critical mass, and more data scientists are involved with experimentation.
Figure 1: current adoption of big data
Source: https://blog.quandl.com/top-5-trends-in-alternative-data
While some alternative data sets have not necessarily lived up to the hype so far, it has become clear that early adopters will be in a very advantageous position compared to those playing catch up, and we are seeing a large uptick in activity in this area as we see an explosion of alternative data providers (see figure 3).
Figure 3: Growth in alternative data providers as an indicator of interest in the field
Employing such methods comes with a host of challenges, still being worked out by the industry:
- Incorporating alternative data and linking to other internal data sources can prove a costly and manual challenge, including the infrastructure necessary for very large datasets
- With the huge increase in providers and variety of data, choosing which data to test can be difficult
- Finding signal in the data, and ensuring that this signal is worth the expense employed
- Finding the specialist skills, with sufficient data science and financial skills to understand all aspects of the exercise
- The perception that data could be regarded as material non-public in the case of exclusively bought datasets, amongst other possible legal issues that need some consideration
It is a very interesting and exciting time in this area of the industry. With all the hype of artificial intelligence and machine learning over the past few years, we are currently seeing all industries take a more pragmatic approach in deciding which use cases they want to test, and proving the value of those chosen use cases. Within investment management, this is clearly a topic of vital interest as investment managers urgently seek alpha at the cusp of a potential downturn, and those who have invested and experimented sufficiently will be clear winners over the next decade.
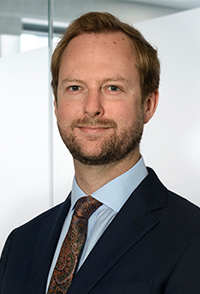
Andrew Morgan is a Senior Manager at Deloitte working in the area of innovation and data science across financial services