Author: Chris Vryonides
The first of this three-part series, Chris Vryonides, Director at AI consultancy Cognosian, examines how machine learning can be used in finance
In "A Mathematician's Apology", GH Hardy famously wrote: "Exposition, criticism, appreciation, is work for second-rate minds".
To discuss the distinction between artificial intelligence (AI), machine learning (ML) and (heaven help us) cognitive computing, feels third rate 1 at best. There will, for better or worse, come a day where genuine AI is upon us, and AI will mean precisely that, with ML demoted to describing stat bashing with big computers and bigger data. If, indeed, it does not mean that already.
While this has implications for how these methods are marketed, we will not get too bogged down in questions like that and ask instead: “Given the phenomenal achievements of ML in other industries, can it be applied in finance?”
Note that there are related areas – credit scoring, fraud detection etc. where ML methods have been successfully applied for some time. Our focus here is more on the markets side of matters.
The need for ML in financial markets
Considering the buy-side first: the quant investing space has become crowded. Increasing capital has been allocated to ever more similar themes and strategies, leading to alpha decay.
On the sell side, market makers are faced with expanding volumes of data and client sophistication; keeping on top of multiple liquidity sources and adjusting systems to deal with difficult customers is ripe for automation and there is a clear requirement to predict market moves (short-term alpha) to enhance both liquidity provision and algorithmic execution services.
Regardless of which side we are on, market relationships that were once clear cut and linear morph into higher order, non-linear behavior as the more obvious opportunities are exploited.
While non-linear methods predate ML, we can argue that ML has popularised their adoption as many ML model setups are implicitly non-linear.
Secondly, ML is clearly the only game in town if we wish to exploit alternative datasets, say tweet sentiment or satellite imagery of retail centre car parks, in some systematic fashion.
Normative economics has clearly had its day; in the search for new, ideally (and implicitly) uncorrelated sources of alpha, where can we turn to, if not ML?
Hype and practice
Hype abounds in our industry, with the biggest institutions often being the biggest offenders. Much of it is marketing, for fear of being left behind competitors purporting to do AI when they are doing nothing of the sort. Slightly less disingenuous is the practice of presenting techniques which have been stalwarts of the statistical community for decades as the latest and greatest innovation.
Of course, AI researchers are guilty of this too, at least from a marketing standpoint.
Deep learning and friends
To read news articles on AI in the last few years, we might think the field comprised little more than the application of deep learning – i.e. deep neural networks.
Let's take a step back; historically, much of the real-world application of AI/ML involved feature engineering - hand crafting functions of raw data, to make the task of simple ML models easier and also enabling the incorporation of (human) expert knowledge.
In the early days of image recognition, images were preprocessed to identify features such as edges and corners, with these then submitted to the recognition algorithm proper.
In the financial setting, technical indicators are nothing more than simple features derived from price data. Again, the idea is to make the eventual algorithm's job easier - in many fields, a linear model will do the job if given well-constructed features. As a bonus, the model can remain interpretable.
Does this count as ML? Arguably, we don’t consider this a particularly enlightening discussion.
Returning to deep learning - the idea here is that by adding more layers 2 to a neural network, it can capture more complicated relationships in the raw input data, obviating much trial and effort in feature engineering. The downside is that many more data are required to train the network, with a concomitant increase in hardware requirements (and, as we shall see in part 3, a danger of overfitting, aka out-of-sample model failure).
This volume of data, and hardware, have only become available recently, but interesting variations aside (e.g. generative adversarial nets) there is nothing drastically new about deep neural networks since artificial neuron models were proposed in 1943. There is also much anecdotal evidence that original attempts at deep learning failed because researchers got their sums wrong.
Consider also Google DeepMind's AlphaZero, where a machine learns to play board games at a level exceeding world champion performance by repeatedly playing against itself. Make no mistake, this is a genuinely impressive achievement (only 10 years ago many AI experts considered it decades away), yet the idea goes back at least as far as 1983; see if you can spot this one:
The point here is that there aren’t a huge number of radically new concepts; ML is as prey to the vicissitudes of fashion as any other field. The recent successes of ML are due to technological advances, an explosion in data and, most importantly: collaboration 3.
Even so…
Nonetheless, there are market participants successfully applying genuine ML techniques to provide both investment decision support or to directly trade markets. Most of these are found at smaller shops, such as hedge funds that aren't lumbered with the secondary challenges foisted on their sell-side cousins.
------------------------------------------------------------------------
1 On the other hand, anyone in this field who does not oft feel overwhelmed keeping up with the volume of research being published daily is probably not quite as smart as he thinks.
2 I once heard of an argument concerning the minimum number of layers a neural network had to have to be considered "deep". When both sides eventually settled on "three", they clearly missed the point: the network is deep when it learns features for us. That said, they did establish a useful lower bound on the length of a piece of string for posterity, for which we can all be grateful.
3 There is little as far-fetched on the big screen as the sight of an artificial general intelligence created by a solitary genius.
---------------------------------------------------------------------------
Read the second article of the series Read the third article of the series
-----------------------------------------------------------------------------
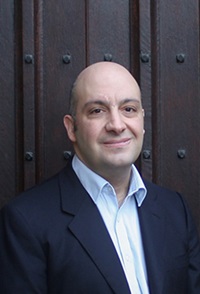
He has over 20 years prior experience of quantitative finance in both bulge bracket banking and investment management and has been applying machine learning and statistical methods since 2008.
This article was produced on behalf of the CFA UK Fintech AI & Machine Learning working group.